In today’s competitive retail landscape, relevance at scale is the name of the game, and machine learning is here to win it for brands. Machine learning technology has the ability to dig through huge data sets to uncover insights about trends, preferences, and even future predictions about what would be the next best interaction between consumers and companies.
We’ve picked out three brands using machine learning to better connect with shoppers. Here’s how they’re putting big data into action to personalize experiences across channels.
1. Under Armour
Under Armour integrated machine learning into Record, the apparel brand’s fitness app. By tracking a user’s health based on data from several sources (third-party apps, smart watches, data entered by users directly, and more), the app is able to provide personalized diet and exercise advice to users.
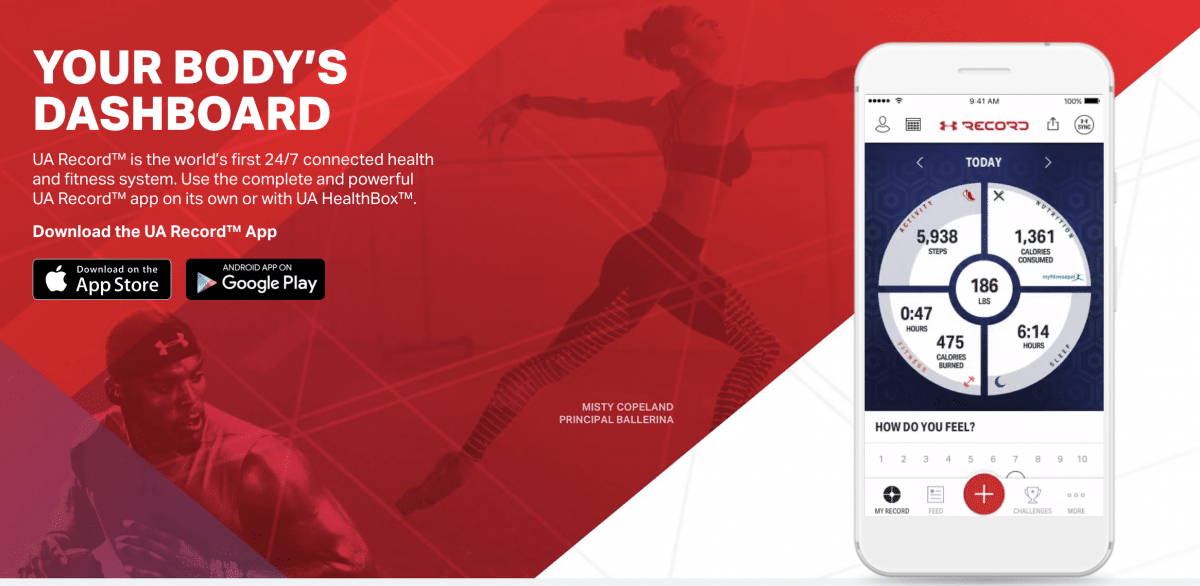
“It will base its coaching on the results of other people who have similar health/fitness profiles, as well as data pulled from things like nutritional databases, physiological, and behavioral data,” wrote Business Insider. The app also factors in things like steps, nutrition, sleep patterns, and workout stats, such as heart rate, pace, distance, and calorie burn.
Effectively a mobile personal trainer, the UA app is a valuable, customized resource that users can use with just a few simple taps. The more you use it, the more it gets to know you, and the better it can customize recommendations to your particular goals.
2. Mazda
Mazda utilized machine learning to find the right influencers to promote a new car, the CX-5, at the SXSW festival in Austin.
The Japanese automotive brand worked with Influential, a company that leverages IBM’s Watson AI technology to identify artistic extroverts (the perfect personas to connect with the festival’s fan base) on social media.
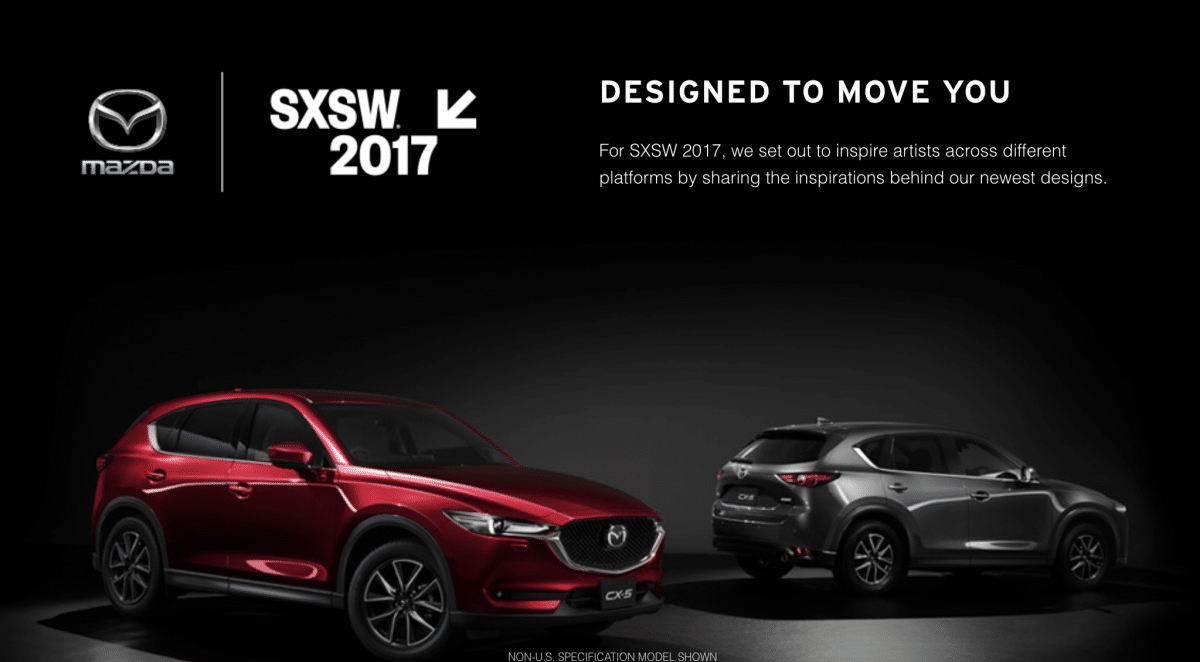
Machine learning technology scanned posts across social media networks, looking for the right people with the right indicators, such as exclamation points and emojis.
For SXSW, four people were selected to cruise around Austin in the CX-5 and hang out in a branded Mazda Studio, then post about the experience on Twitter, Instagram, and Facebook.
This data-centric approach to influencer marketing allowed Mazda personalize content to better engage with a very specific crowd, one that they knew leaned more creative — given that SXSW celebrates music, film, and tech, among other innovative ideas — than a general audience segment.
(Learn more: Would you survive in a tech-less world? Find out with this interactive quiz.)
3. The North Face
The North Face uses a digital personal assistant, Fluid’s Expert Personal Shopper (XPS), to help make recommendations to online shoppers, build out profiles based on their interests, and create a personalized user experience.
XPS extends the outdoor clothing retailer’s welcoming and helpful in-store reputation to the digital realm. By using machine learning, the brand can simultaneously collect data to inform website iterations and future products.
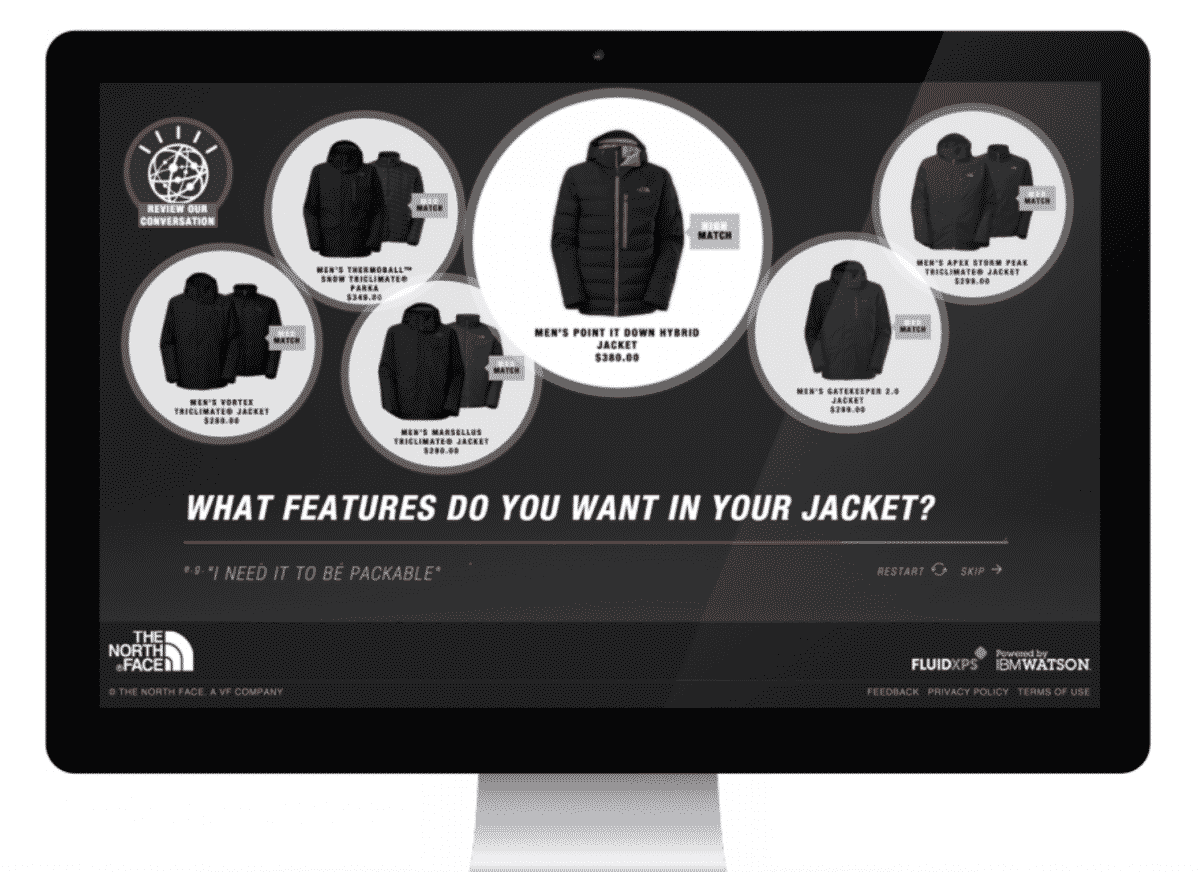
The North Face also delivers a highly personalized shopping experience called “Shop with IBM Watson” to website users.
After downloading the mobile app, shoppers can speak into their smartphones to access the technology. Just as a salesperson can help customers select the best option for their needs, this virtual assistant asks users questions, learns from their responses, and offers relevant products to satisfy their preferences.
The ROI of Machine Learning
The predictive nature of machine learning is what makes it such a powerful tool for commerce marketers. ML allows brands to use past and present customer data to project future behavior and trends.
On the sales side, machine learning models can predict the most relevant time to offer products (e.g. when a shopper is most likely to buy seasonal items). In terms of operations, machine learning can help retailers estimate how much inventory to stock during peak sales times (like Black Friday, Cyber Monday, etc) compared to the rest of the year.
As a result, machine learning models help cut back on unnecessary spend (like wasted ad dollars and leftover products) while optimizing marketing efforts to anticipate customer needs. Taken together, this leads to increased revenue and higher profit margins for brands.
To learn more about the ways machine learning is changing retail, download our eBook.